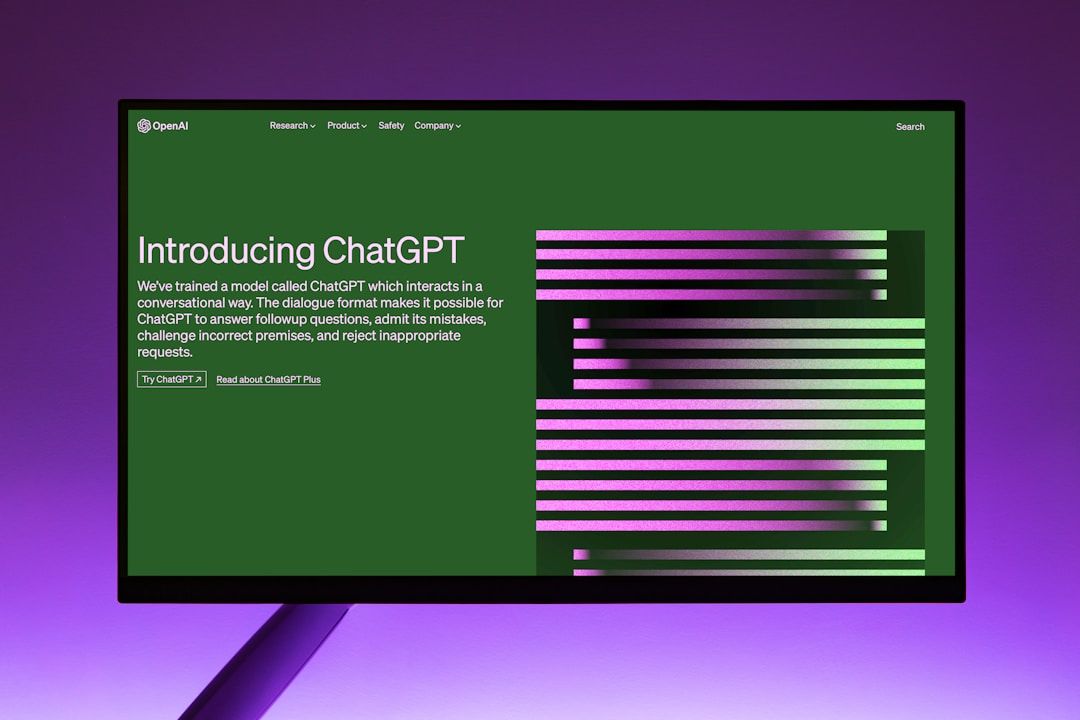
In the rapidly evolving landscape of artificial intelligence, generative AI has emerged as a transformative force, revolutionising various industries with its innovative capabilities. As we move further into 2024, it is essential for professionals and businesses to understand the latest trends and applications of generative AI to harness its full potential.
Understanding Generative AI
Generative AI refers to a class of artificial intelligence systems that can create new content, such as text, images, audio, and video, by learning from existing data. Unlike traditional AI models that focus on analysing and interpreting data, generative AI models use sophisticated algorithms to generate original content that mimics human creativity. Key technologies driving generative AI include Generative Adversarial Networks (GANs), Variational Autoencoders (VAEs), and transformer-based models like GPT-4.
Current Applications of Generative AI
- Media and Entertainment Generative AI has made significant inroads in the media and entertainment industry. Companies like Runway have developed generative video models capable of producing high-quality video content. These advancements allow filmmakers to create realistic special effects, de-age actors, and produce multilingual lip-syncs with unprecedented accuracy. Major studios, including Paramount and Disney, are incorporating these tools into their production pipelines to enhance visual storytelling and streamline post-production processes (MIT Technology Review).
- Healthcare In healthcare, generative AI is transforming diagnostics, personalised medicine, and medical research. AI models can analyse medical images, such as X-rays and MRIs, to detect anomalies with high precision. Additionally, generative AI is used to simulate complex biological processes and predict the effects of new drugs, significantly accelerating the drug discovery process. This technology also facilitates the creation of synthetic medical data, which can be used to train AI models without compromising patient privacy (World Economic Forum) (TechRepublic).
- Finance The finance industry is leveraging generative AI to enhance fraud detection, automate trading strategies, and personalise customer experiences. AI-driven algorithms can analyse vast amounts of financial data to identify fraudulent activities in real-time. Generative models are also used to create synthetic data that helps financial institutions train their AI systems without exposing sensitive information. Moreover, personalised financial advice and product recommendations are generated based on individual customer profiles, improving customer engagement and satisfaction (TechRepublic).
- Marketing and Content Creation Generative AI is revolutionising marketing by automating content creation and enhancing customer interactions. Tools like GPT-4 can generate high-quality written content, including articles, social media posts, and ad copy, tailored to specific audiences. AI-generated images and videos are used in advertising campaigns to create visually appealing and engaging content. This automation not only saves time but also ensures consistency and creativity in marketing efforts (IBM — United States) (Source).
Technological Advancements in Generative AI
Recent technological advancements have made generative AI more accessible and efficient. Techniques such as Low Rank Adaptation (LoRA) and Quantisation have significantly reduced the computational resources required for training and fine-tuning AI models. LoRA, for instance, allows the injection of trainable layers into pre-trained models, speeding up the fine-tuning process and reducing memory usage. Quantisation, on the other hand, lowers the precision of model data points, thereby enhancing the speed and efficiency of AI models (IBM — United States).
The development of smaller, more efficient language models (SLMs) is another noteworthy trend. These models, despite their reduced size, perform comparably to large language models (LLMs) in specific tasks, making AI more affordable and accessible. Researchers are increasingly focusing on using high-quality, curated training data to optimise the performance of SLMs, challenging the notion that larger models are inherently better (Source).
Best Practices for Implementing Generative AI
- Ethical Considerations The use of generative AI raises important ethical questions, particularly concerning data privacy, bias, and misinformation. It is crucial to implement robust ethical guidelines to ensure that AI systems are developed and deployed responsibly. This includes conducting thorough audits of AI models to detect and mitigate biases, ensuring transparency in AI decision-making processes, and adhering to data privacy regulations (TechRepublic).
- Data Management Effective data management is vital for the success of generative AI projects. Organisations should invest in high-quality data collection and curation processes to train AI models accurately. Maintaining diverse and representative datasets helps minimise biases and improve the generalisability of AI systems. Additionally, leveraging synthetic data generated by AI can augment real-world data, providing a richer training environment while safeguarding privacy (World Economic Forum).
- Collaboration and Integration Collaboration between industry and academia is essential for advancing generative AI technologies. Joint research initiatives can lead to the development of innovative AI models and applications. Integrating AI tools into existing workflows requires careful planning and coordination across different departments within an organisation. Ensuring that employees are adequately trained and equipped to work with AI systems is also critical for successful implementation (Source) (TechRepublic).
- Continuous Monitoring and Improvement Generative AI models should be continuously monitored and updated to maintain their relevance and accuracy. Implementing feedback loops allows AI systems to learn from new data and adapt to changing conditions. Regularly evaluating the performance of AI models against standardised benchmarks can help identify areas for improvement and ensure that the systems remain effective and reliable (World Economic Forum).
Conclusion
Generative AI is a powerful tool that holds immense potential for transforming various industries. By understanding the latest trends and applications, businesses can leverage this technology to drive innovation, improve efficiency, and create value. However, it is essential to approach generative AI with a focus on ethical considerations, robust data management, and continuous improvement to ensure its responsible and effective use. As generative AI continues to evolve, staying informed and adaptable will be key to harnessing its full potential and achieving long-term success.
Are you ready to harness the power of generative AI and drive innovation within your organisation? Stay ahead of the curve by exploring our comprehensive courses and resources at Duco Digital Training. Whether you’re looking to deepen your understanding of AI ethics, learn about the latest advancements in AI technology, or integrate AI into your business strategy, our expert-led programs are designed to equip you with the skills and knowledge you need to succeed.
Join us today and transform your digital journey with Duco Digital Training.
Explore Our Courses | Contact Us | Subscribe to Our Newsletter
Stay informed, stay competitive, and lead the way in the digital era. Your future in AI starts here!